2 Data preprocessing
2.1 Data import: GPS and raster data
I start by importing GPS data of adults only (without individuals from the Jacksonville zoo), in the form of trajectories (ltraj
) that is converted to point data (SpatialPointsDataFrame
) and projected to Lat/Long:
library("hab")
load("Data/wost-ad-ltraj.RData")
library("rgdal")
wost <- hab::ltraj2spdf(trajad, strict = FALSE, proj4string = CRS("+init=epsg:32617"))
wost$id <- factor(wost$id)
wost <- subset(wost, !(id %in% c(407680, 429200, 476550, 721310, 721320)))
wost <- spTransform(wost, CRS("+proj=longlat +datum=WGS84"))
summary(wost)
## Object of class SpatialPointsDataFrame
## Coordinates:
## min max
## x -91.45783 -78.01650
## y 25.08267 34.60033
## Is projected: FALSE
## proj4string :
## [+proj=longlat +datum=WGS84 +ellps=WGS84 +towgs84=0,0,0]
## Number of points: 445638
## Data attributes:
## date dx dy
## Min. :2005-07-29 07:00:00 Min. :-90334.77 Min. :-127323.69
## 1st Qu.:2007-09-28 19:00:00 1st Qu.: -30.13 1st Qu.: -19.90
## Median :2009-07-09 17:00:00 Median : 0.00 Median : 0.00
## Mean :2009-04-11 15:12:47 Mean : 5.56 Mean : -12.55
## 3rd Qu.:2010-10-29 20:00:00 3rd Qu.: 31.28 3rd Qu.: 19.58
## Max. :2011-12-31 18:00:00 Max. :133219.05 Max. : 148420.54
## NA's :36011 NA's :36011
## dist dt R2n
## Min. : 0.00 Min. :3600 Min. : 0
## 1st Qu.: 16.76 1st Qu.:3600 1st Qu.: 108970
## Median : 49.76 Median :3600 Median : 9802756
## Mean : 1544.56 Mean :4777 Mean : 24050154883
## 3rd Qu.: 639.01 3rd Qu.:7200 3rd Qu.: 1192219334
## Max. :150312.14 Max. :7200 Max. :1005475966130
## NA's :36011 NA's :22863
## abs.angle rel.angle id burst
## Min. :-3.14 Min. :-3.14 572931 : 29866 414590 : 21736
## 1st Qu.:-1.57 1st Qu.:-1.57 414590 : 21736 414240 : 18336
## Median : 0.00 Median : 0.15 414240 : 18336 414580 : 14595
## Mean : 0.09 Mean : 0.18 721290 : 16319 416110 : 14110
## 3rd Qu.: 1.57 3rd Qu.: 2.04 723560 : 15763 407700 : 13064
## Max. : 3.14 Max. : 3.14 414580 : 14595 414260 : 12473
## NA's :110418 NA's :143287 (Other):329023 (Other):351324
## gid elevation day
## Min. :148996 Min. :-999.0 Min. : 429
## 1st Qu.:293034 1st Qu.: 3.0 1st Qu.:1220
## Median :456866 Median : 10.0 Median :1870
## Mean :442556 Mean :-173.2 Mean :1781
## 3rd Qu.:584878 3rd Qu.: 28.0 3rd Qu.:2347
## Max. :701216 Max. :2032.0 Max. :2775
## NA's :22873
wost2 <- droplevels(wost@data)
length(unique(wost2$id))
## [1] 61
table(wost2$id)
##
## 407700 407710 414240 414250 414260 414270 414580 414590 414600 414610
## 13064 1463 18336 5676 12473 2523 14595 21736 8671 628
## 416100 416110 425120 429190 475171 475180 475192 475220 475241 475270
## 651 14110 5093 11316 2683 11720 650 2137 19 4867
## 475282 475284 475302 475322 519770 519790 519800 519810 572661 572662
## 1790 1245 2729 505 8973 7306 8909 7876 3998 3839
## 572671 572710 572741 572742 572743 572781 572800 572811 572931 572951
## 1083 10281 346 2268 3202 4386 1098 5881 29866 7911
## 572981 572991 721290 721300 723560 723570 723580 723600 723610 809320
## 4614 8626 16319 9859 15763 2824 2134 2910 7459 5638
## 809330 809340 910200 910210 910220 910230 910240 992250 992270 992280
## 805 9165 13990 14474 14269 14105 3372 9188 7607 8761
## 992290
## 7853
summary(as.numeric(table(wost2$id)))
## Min. 1st Qu. Median Mean 3rd Qu. Max.
## 19 2523 5881 7306 10280 29870
sd(as.numeric(table(wost2$id)))
## [1] 6036.537
summary(unlist(by(wost2, wost2$id, function(k) difftime(max(k$date), min(k$date),
units = "days"))))
## Min. 1st Qu. Median Mean 3rd Qu. Max.
## 1.25 247.50 527.50 649.10 972.30 2326.00
sd(unlist(by(wost2, wost2$id, function(k) difftime(max(k$date), min(k$date),
units = "days"))))
## [1] 562.5885
I also import environmental data (from files). All rasters are combined in a RasterBrick
, which is then masked using a Minimum convex polygon (from adehabitatHR
) of every relocations enlarged by a one-cell buffer:
library("raster")
janPrec <- raster("Data/Consensus_rasters/jan_prec.img")
janTemp <- raster("Data/Consensus_rasters/jan_temp.img")
febPrec <- raster("Data/Consensus_rasters/feb_prec.img")
febTemp <- raster("Data/Consensus_rasters/feb_temp.img")
marPrec <- raster("Data/Consensus_rasters/mar_prec.img")
marTemp <- raster("Data/Consensus_rasters/mar_temp.img")
aprPrec <- raster("Data/Consensus_rasters/apr_prec.img")
aprTemp <- raster("Data/Consensus_rasters/apr_temp.img")
mayPrec <- raster("Data/Consensus_rasters/may_prec.img")
mayTemp <- raster("Data/Consensus_rasters/may_temp.img")
junPrec <- raster("Data/Consensus_rasters/jun_prec.img")
junTemp <- raster("Data/Consensus_rasters/jun_temp.img")
julPrec <- raster("Data/Consensus_rasters/jul_prec.img")
julTemp <- raster("Data/Consensus_rasters/jul_temp.img")
augPrec <- raster("Data/Consensus_rasters/aug_prec.img")
augTemp <- raster("Data/Consensus_rasters/aug_temp.img")
sepPrec <- raster("Data/Consensus_rasters/sep_prec.img")
sepTemp <- raster("Data/Consensus_rasters/sep_temp.img")
octPrec <- raster("Data/Consensus_rasters/oct_prec.img")
octTemp <- raster("Data/Consensus_rasters/oct_temp.img")
decPrec <- raster("Data/Consensus_rasters/dec_prec.img")
decTemp <- raster("Data/Consensus_rasters/dec_temp.img")
novPrec <- raster("Data/Consensus_rasters/nov_prec.img")
novTemp <- raster("Data/Consensus_rasters/nov_temp.img")
(rast <- brick(janPrec, janTemp, febPrec, febTemp, marPrec, marTemp, aprPrec,
aprTemp, mayPrec, mayTemp, junPrec, junTemp, julPrec, julTemp, augPrec,
augTemp, sepPrec, sepTemp, octPrec, octTemp, novPrec, novTemp, decPrec,
decTemp))
## class : RasterBrick
## dimensions : 855, 2156, 1843380, 24 (nrow, ncol, ncell, nlayers)
## resolution : 0.167, 0.167 (x, y)
## extent : -180, 180.052, -59.1665, 83.6185 (xmin, xmax, ymin, ymax)
## crs : NA
## source : /tmp/Rtmp4OILip/raster/r_tmp_2019-10-30_173244_13787_41238.grd
## names : jan_prec, jan_temp, feb_prec, feb_temp, mar_prec, mar_temp, apr_prec, apr_temp, may_prec, may_temp, jun_prec, jun_temp, jul_prec, jul_temp, aug_prec, ...
## min values : 0.00, -51.45, 0.00, -47.45, 0.00, -44.55, 0.00, -35.75, 0.00, -19.80, 0.00, -13.55, 0.00, -10.85, 0.00, ...
## max values : 808.90, 33.10, 714.15, 32.45, 689.05, 32.25, 632.15, 33.90, 965.00, 35.80, 2136.30, 38.35, 2123.25, 38.20, 1538.70, ...
library("rgeos")
sa <- mcp(wost, percent = 100)
rast <- crop(rast, gBuffer(sa, width = 0.167))
rast <- mask(rast, gBuffer(sa, width = 0.167))
The result is something like this (using temperatures in January as an example)
library("sf")
library("maps")
states <- st_as_sf(map("state", plot = FALSE, fill = TRUE))
rastDF <- data.frame(rasterToPoints(rast))
library("cowplot")
library("viridis")
ggplot() + geom_raster(data = rastDF, aes_string(x = "x", y = "y", fill = names(rastDF)[4])) +
geom_sf(data = states, fill = NA, size = 0.2, colour = gray(0.2)) + geom_point(data = data.frame(wost),
aes(x = x, y = y), shape = 20, size = 0.8, stroke = 0, colour = "gray") +
coord_sf(xlim = c(-92, -77.5), ylim = c(24.5, 35), expand = FALSE) + scale_fill_viridis(end = 0.8,
alpha = 1, option = "plasma") + labs(x = "Latitude", y = "Longitude", fill = expression(`Temperature `(degree ~
C))) + theme(legend.position = "top", legend.justification = "center") +
guides(fill = guide_colourbar(barwidth = 15, barheight = 1, title.theme = element_text(size = 26)))
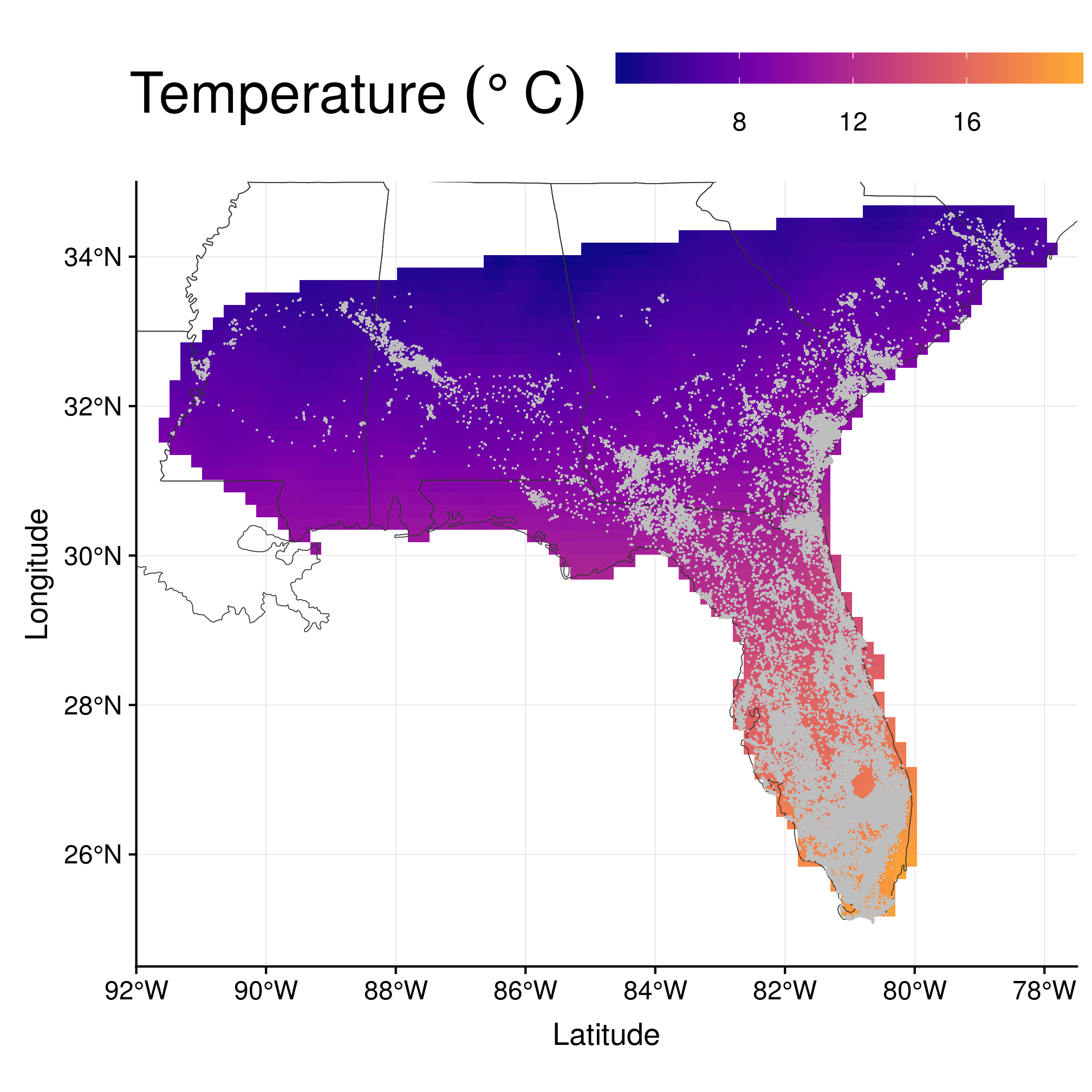
Figure 2.1: Temperature in January.
2.2 Environmental intersection
We first look at the frequency of data for each month:
library("lubridate")
wost$month <- month(wost$date)
table(wost$month)
##
## 1 2 3 4 5 6 7 8 9 10 11 12
## 28931 26156 30298 33741 40787 38467 42136 44483 43625 42663 38409 35942
plot(table(wost$month), ylab = "Frequency", xlab = "Month")
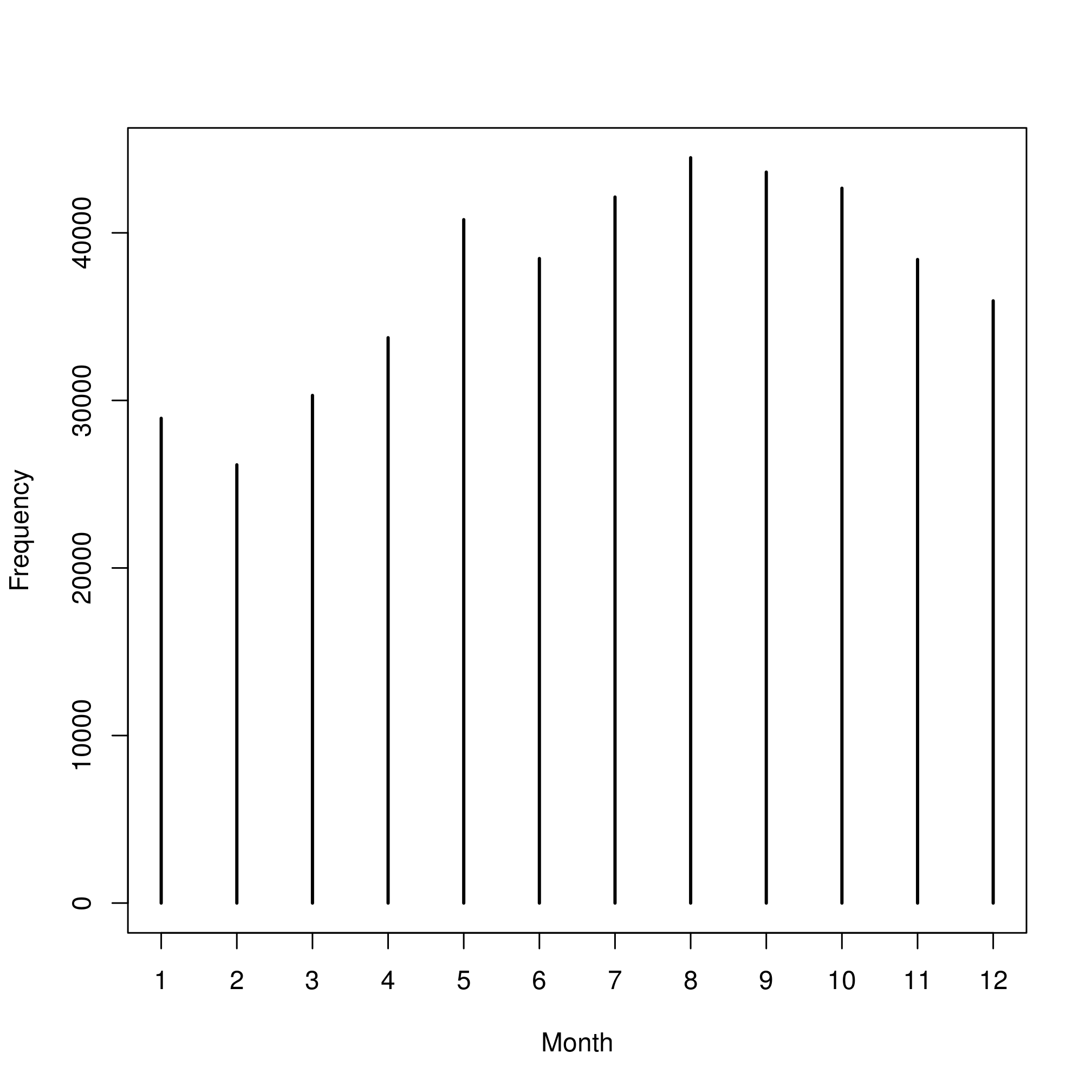
Figure 2.2: Frequency of data per month.
We then intersect the GPS data to the precipitation and temperature layers, using the month number to identify the correct layer:
wost$prec <- ifelse(wost$month == 1, extract(janPrec, wost),
ifelse(wost$month == 2, extract(febPrec, wost),
ifelse(wost$month == 3, extract(marPrec, wost),
ifelse(wost$month == 4, extract(aprPrec, wost),
ifelse(wost$month == 5, extract(mayPrec, wost),
ifelse(wost$month == 6, extract(junPrec, wost),
ifelse(wost$month == 7, extract(julPrec, wost),
ifelse(wost$month == 8, extract(augPrec, wost),
ifelse(wost$month == 9, extract(sepPrec, wost),
ifelse(wost$month == 10, extract(octPrec, wost),
ifelse(wost$month == 11, extract(novPrec, wost),
extract(decPrec, wost))))))))))))
wost$temp <- ifelse(wost$month == 1, extract(janTemp, wost),
ifelse(wost$month == 2, extract(febTemp, wost),
ifelse(wost$month == 3, extract(marTemp, wost),
ifelse(wost$month == 4, extract(aprTemp, wost),
ifelse(wost$month == 5, extract(mayTemp, wost),
ifelse(wost$month == 6, extract(junTemp, wost),
ifelse(wost$month == 7, extract(julTemp, wost),
ifelse(wost$month == 8, extract(augTemp, wost),
ifelse(wost$month == 9, extract(sepTemp, wost),
ifelse(wost$month == 10, extract(octTemp, wost),
ifelse(wost$month == 11, extract(novTemp, wost),
extract(decTemp, wost))))))))))))
Note that the correlation between temperature and precipitation in wood stork use data is pretty high.
cor(wost$prec, wost$temp, use = "complete.obs")
## [1] 0.7965986
plot(wost$prec, wost$temp, pch = 20, xlab = "Precipitation", ylab = "Temperature")
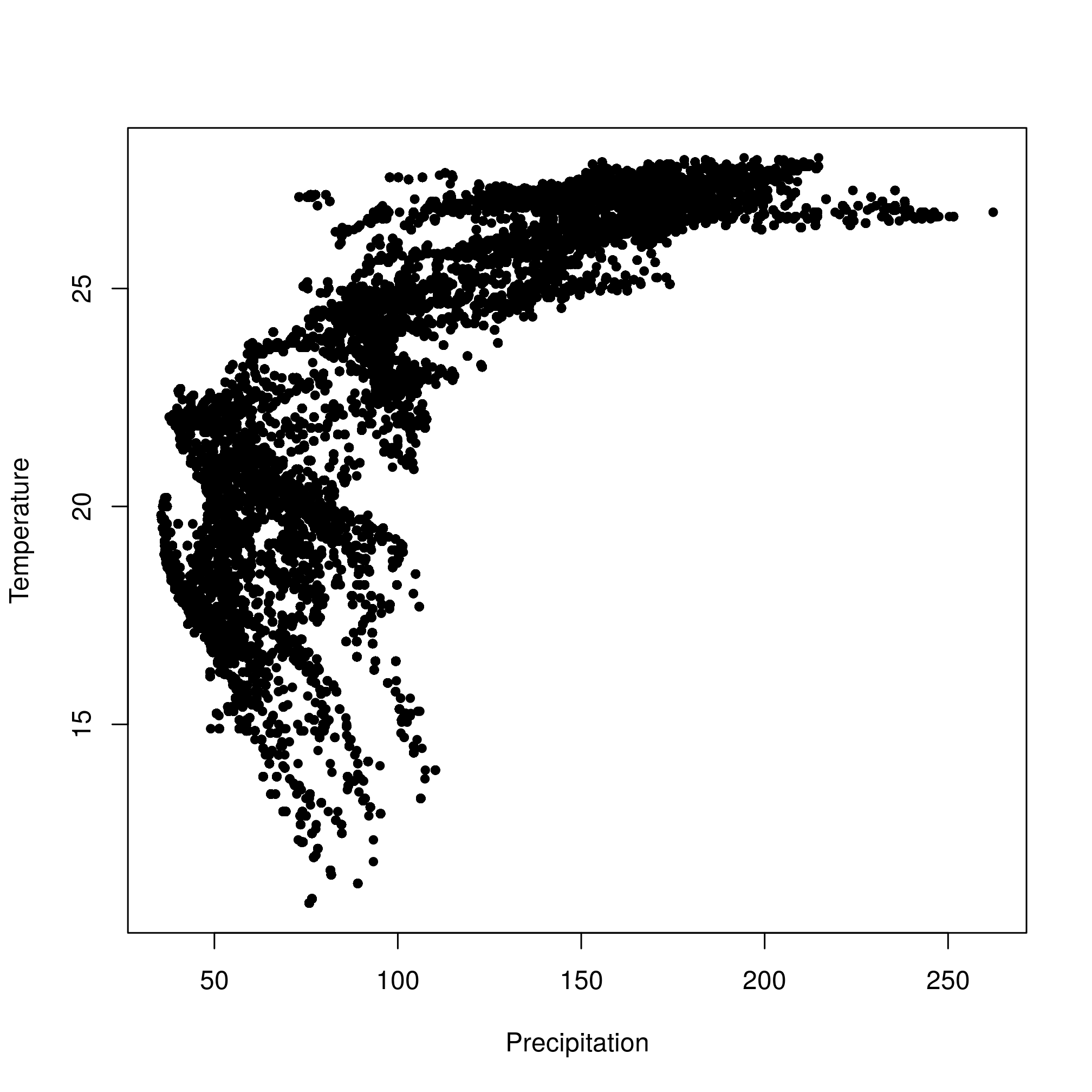
Figure 2.3: Correlation between precipitation and temperature.
2.3 Sampling the point data
In this section, I will sample GPS data to ensure that each individual is represented equally in each month. I first break down the data into a list with one element per month. Over this list, i.e. for each month, individuals with less than 100 locations are removed, and one random location of a random individual (with replacement) is chosen 5000 times, to give each individual the same weight in the sampled data:
table(wost$id, wost$month)
##
## 1 2 3 4 5 6 7 8 9 10 11 12
## 407680 0 0 0 0 0 0 0 0 0 0 0 0
## 407700 956 856 897 911 1040 950 1325 1371 1211 1257 1295 995
## 407710 0 0 0 0 0 0 323 308 329 342 161 0
## 414240 1488 1328 1486 1490 1579 1595 1633 1638 1551 1555 1453 1540
## 414250 266 311 313 539 637 619 629 693 617 467 290 295
## 414260 947 739 781 1057 1232 1062 1207 1189 1087 1090 1040 1042
## 414270 0 0 0 175 317 320 337 327 308 348 319 72
## 414580 903 855 962 1390 1376 1416 1632 1504 1404 1233 945 975
## 414590 1530 1373 1637 1902 1980 1937 2058 1963 1927 1990 1840 1599
## 414600 620 556 554 872 887 809 801 872 778 728 615 579
## 414610 0 0 0 148 244 236 0 0 0 0 0 0
## 416100 0 0 36 285 330 0 0 0 0 0 0 0
## 416110 1005 883 1061 1269 1546 1250 1336 1348 1113 1163 1075 1061
## 425120 144 132 223 398 466 444 791 692 710 535 374 184
## 429190 890 718 851 847 952 887 1086 1122 1065 983 1013 902
## 429200 0 0 0 0 0 0 0 0 0 0 0 0
## 475171 41 10 279 302 424 491 285 302 174 175 114 86
## 475180 508 680 1148 1112 1206 1020 1210 1087 1090 982 968 709
## 475192 0 0 0 331 319 0 0 0 0 0 0 0
## 475220 94 68 172 242 229 221 217 232 194 208 195 65
## 475241 0 0 0 0 0 0 19 0 0 0 0 0
## 475270 277 238 623 659 670 652 530 476 262 142 217 121
## 475282 0 0 0 0 0 0 356 426 422 434 152 0
## 475284 0 0 0 20 155 150 156 155 150 154 150 155
## 475302 317 336 359 396 313 0 24 241 53 133 275 282
## 475322 0 0 0 0 0 0 0 58 437 10 0 0
## 476550 0 0 0 0 0 0 0 0 0 0 0 0
## 519770 457 411 487 452 703 887 926 945 912 952 895 946
## 519790 467 428 470 439 472 446 617 898 907 917 763 482
## 519800 462 412 475 432 655 907 959 947 914 936 877 933
## 519810 470 440 477 446 438 423 625 949 920 901 889 898
## 572661 213 0 0 365 469 319 465 468 456 455 336 452
## 572662 156 0 0 0 440 444 434 472 459 480 474 480
## 572671 0 0 0 385 454 244 0 0 0 0 0 0
## 572710 712 724 1151 1145 1186 1025 704 641 725 777 723 768
## 572741 0 0 0 0 0 0 0 131 215 0 0 0
## 572742 0 0 0 0 0 0 255 444 449 464 404 252
## 572743 429 384 45 0 427 201 117 214 371 422 250 342
## 572781 444 20 32 439 467 346 456 442 459 439 384 458
## 572800 0 0 115 136 121 194 201 245 86 0 0 0
## 572811 460 407 454 450 455 391 430 151 554 892 780 457
## 572931 2456 2360 2416 2385 2420 2141 2434 2611 2786 2727 2541 2589
## 572951 892 786 468 452 423 268 446 643 899 905 858 871
## 572981 464 396 466 403 408 208 42 481 453 433 442 418
## 572991 797 737 856 745 451 286 463 879 841 875 849 847
## 721290 959 1038 1366 1259 1352 1548 1745 1805 1782 1672 940 853
## 721300 804 864 909 826 684 664 909 900 847 815 781 856
## 721310 0 0 0 0 0 0 0 0 0 0 0 0
## 721320 0 0 0 0 0 0 0 0 0 0 0 0
## 723560 1101 1215 1345 1307 1405 1336 1351 1395 1358 1381 1301 1268
## 723570 0 0 0 120 360 323 355 351 354 368 321 272
## 723580 0 0 0 45 356 298 363 359 339 353 21 0
## 723600 0 0 0 120 361 326 370 363 356 369 360 285
## 723610 471 470 826 768 633 738 696 757 758 559 406 377
## 809320 439 410 461 457 647 465 473 476 452 456 454 448
## 809330 0 0 0 0 135 477 193 0 0 0 0 0
## 809340 891 588 453 434 576 830 909 926 852 900 901 905
## 910200 875 794 955 863 1340 1248 1342 1284 1296 1361 1334 1298
## 910210 913 844 924 887 1353 1309 1364 1377 1357 1406 1367 1373
## 910220 906 811 950 917 1371 1271 1367 1390 1331 1364 1338 1253
## 910230 930 819 935 906 1282 1252 1304 1319 1322 1364 1342 1330
## 910240 0 0 0 0 444 418 458 470 455 483 448 196
## 992250 445 434 460 472 813 923 948 964 942 954 900 933
## 992270 466 439 487 450 676 927 928 956 729 484 441 624
## 992280 431 407 457 441 657 911 922 918 904 916 890 907
## 992290 435 435 476 450 451 414 610 908 903 954 908 909
summary(as.numeric(table(wost$id)))
## Min. 1st Qu. Median Mean 3rd Qu. Max.
## 0 1876 5366 6752 9691 29870
months <- split(wost, wost$month)
for (i in 1:length(months)) months[[i]]$id <- factor(months[[i]]$id)
monthLocs <- lapply(months, function(k) {
k <- nsubset(k, id, 100)
k$id <- factor(k$id)
lt <- split(k, k$id)
set.seed(34138)
sam <- sample(1:length(lt), 5000, replace = TRUE)
return(do.call("rbind", lapply(sam, function(x) {
lt[[x]][sample(1:nrow(lt[[x]]), 1), ]
})))
})
monthLocsAll <- do.call(rbind, monthLocs)
table(monthLocsAll$month)
##
## 1 2 3 4 5 6 7 8 9 10 11 12
## 5000 5000 5000 5000 5000 5000 5000 5000 5000 5000 5000 5000
Here is the result on the map of temperatures in January:
ggplot() + geom_raster(data = data.frame(rasterToPoints(rast)), aes_string(x = "x",
y = "y", fill = names(rast)[4])) + geom_sf(data = states, fill = NA, size = 0.2,
colour = gray(0.2)) + geom_point(data = data.frame(wost), aes(x = x, y = y),
shape = 20, size = 0.8, stroke = 0, colour = "gray") + geom_point(data = subset(data.frame(monthLocsAll),
month == 1), aes(x = x, y = y), shape = 15) + coord_sf(xlim = c(-92, -77.5),
ylim = c(24.5, 35), expand = FALSE) + scale_fill_viridis(end = 0.8, alpha = 1,
option = "plasma") + labs(x = "Latitude", y = "Longitude", fill = expression(`Temperature `(degree ~
C))) + theme(legend.position = "top", legend.justification = "center") +
guides(fill = guide_colourbar(barwidth = 15, barheight = 1, title.theme = element_text(size = 26)))
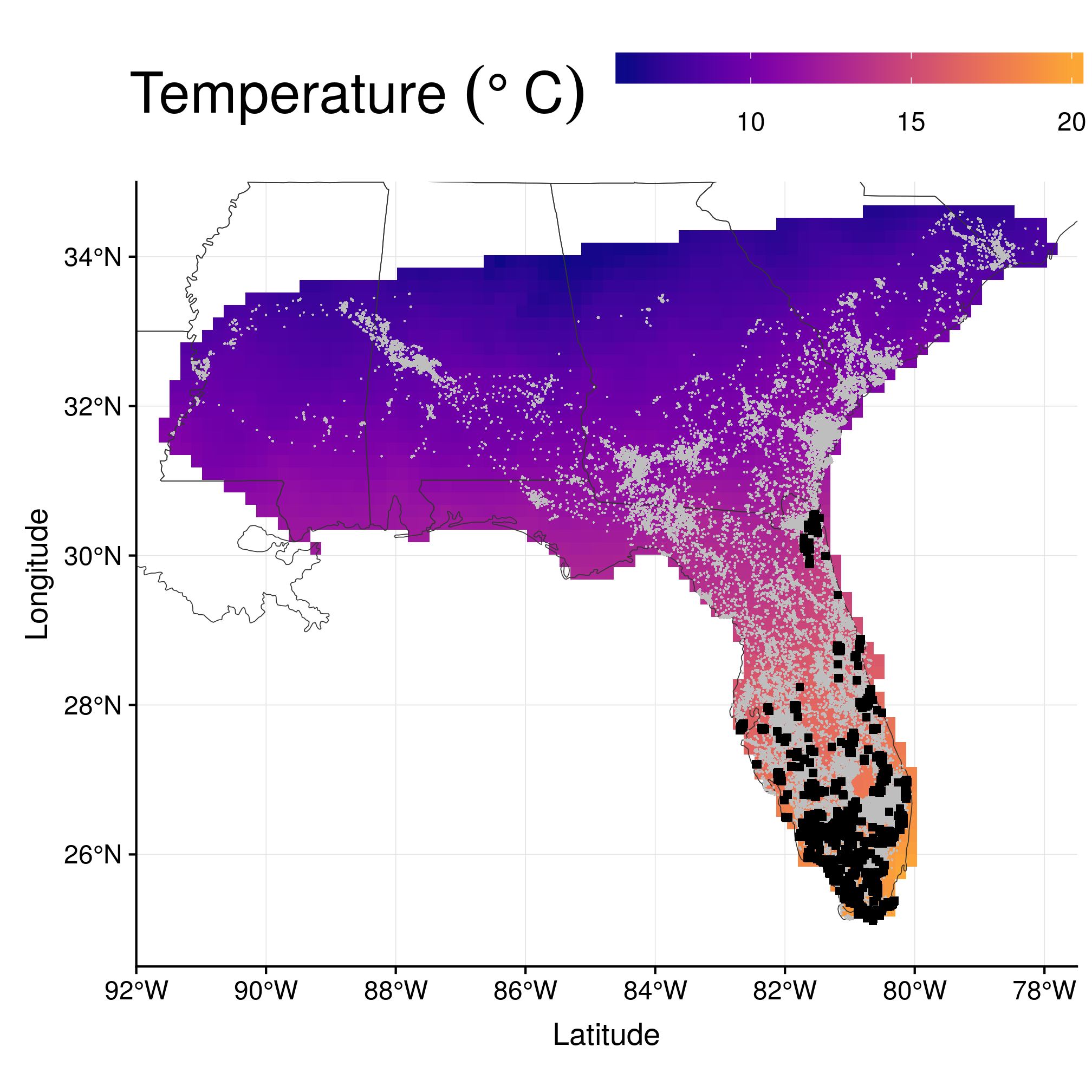
Figure 2.4: Wood stork location data (blue dots), with January temperatures in the background and January locations in black.